XAI: Understanding how machines make decisions
07 July 2021
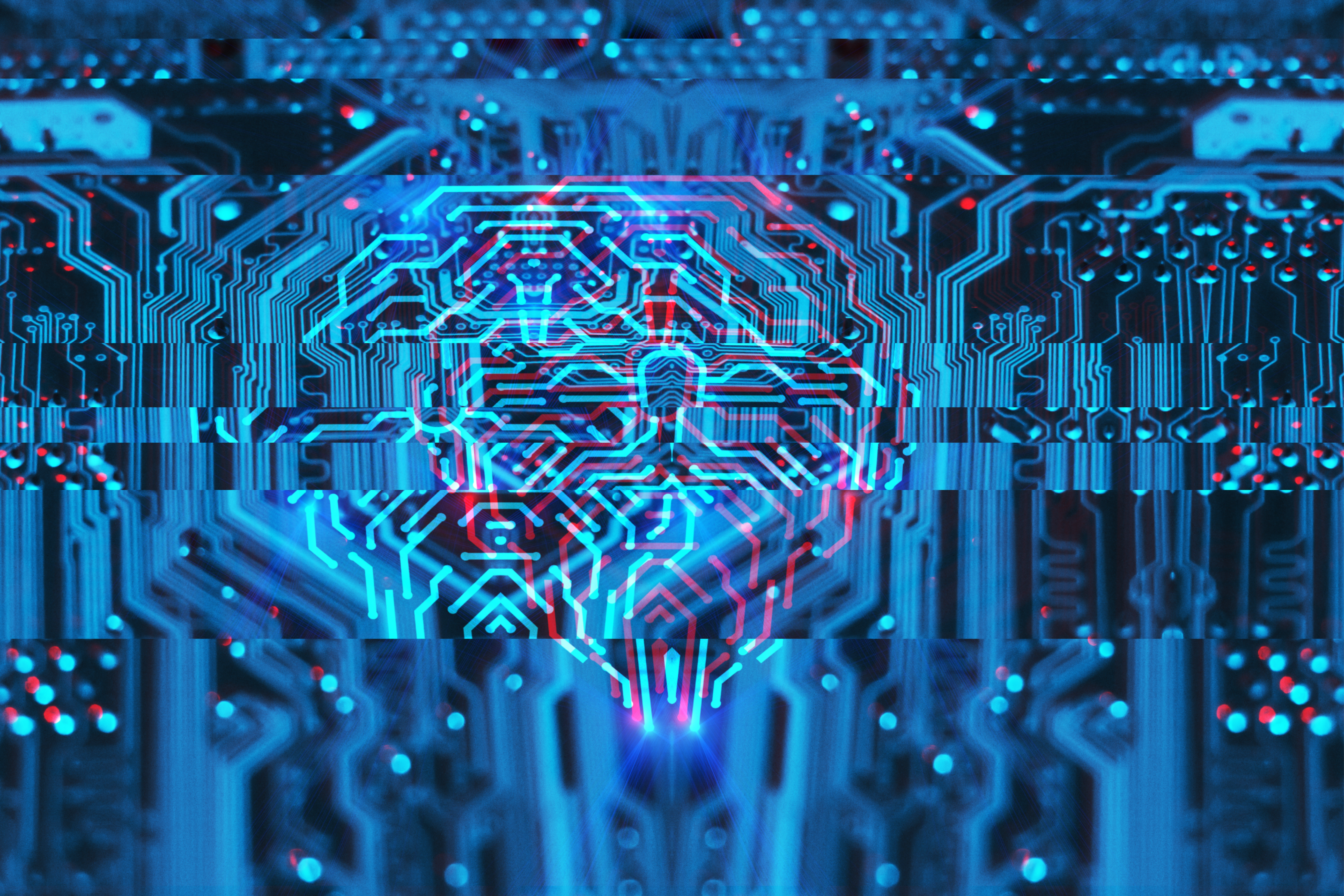
XAI: Explainable artificial intelligence.
As artificial intelligence (AI) moves into our economy and our lives, we need to understand how computers make decisions. A team of Luxembourg researchers are working on making artificial intelligence understandable, known as explainable artificial intelligence (XAI).
Researchers at the Interdisciplinary Centre for Security, Reliability and Trust (SnT) from the University of Luxembourg are developing AI algorithms based on XAI principles. The objective? To make sure that we are aware and in full control of the decisions made by AI.
Explainable artificial intelligence to gain momentum
Machine learning is becoming increasingly important. While such algorithms can give virtually perfect accuracy, the decision-making process remains elusive. With the acceleration of AI regulation and increased user awareness, explainability has emerged as a priority.
From a regulatory standpoint, the General Data Protection Regulation (GDPR) includes the right to explanation, whereby an individual can request explanations on the workings of an algorithmic decision produced based on their data.
Additionally, users’ distrust, due to their lack of understanding of the algorithms, may lead to a reluctance to apply complex machine learning techniques. Explainability may come at the expense of accuracy. When faced with a trade-off between explainability and accuracy, industry players may, for regulatory reasons, have to use less accurate models for production systems.
Finally, without explanations, business experts produce justifications for model behaviour on their own. Such a situation is likely to lead to a plurality of explanations, as they conceive contradictory ideas.
Explainability aims to respond to the opacity of the model and its output while maintaining the performance. It gives machine learning models the ability to explain or to present their behaviours in understandable terms to humans.
A human should understand how the software came to a decision
Integrating explainability in production is a crucial yet complex task that the TruX research group at SnT is exploring. As such, the research team is looking to develop AI algorithms following XAI principles to provide solutions to banking processes, in particular.
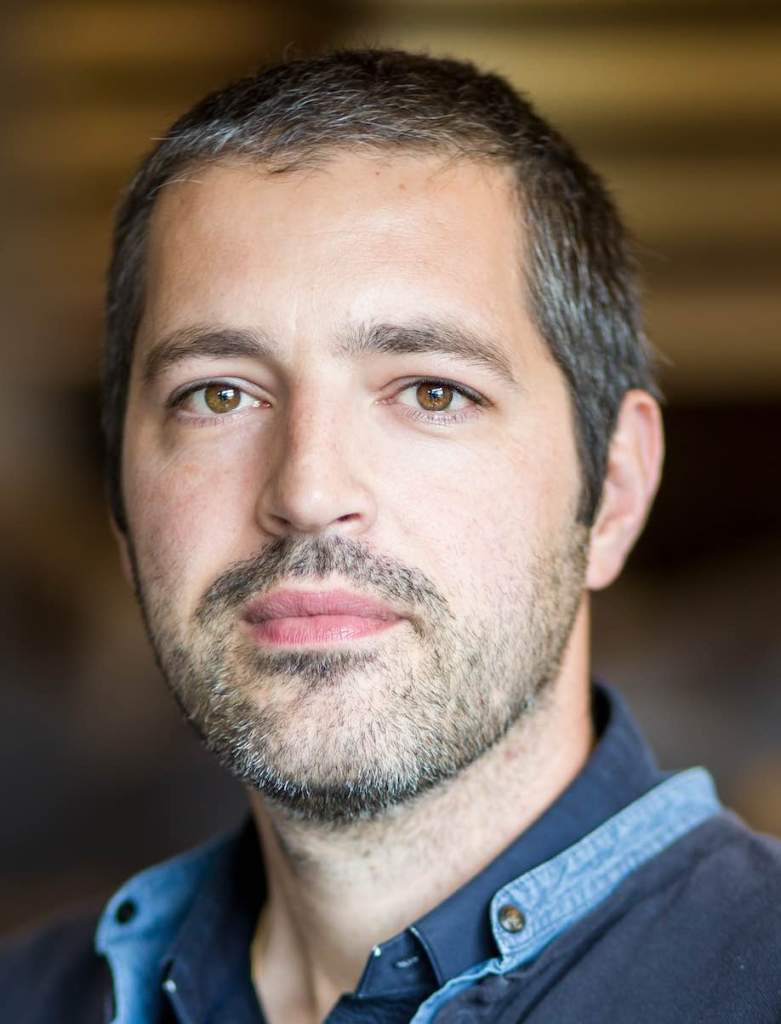
“State-of-the-art frameworks have now outmatched human accuracy in complex pattern recognition tasks. Many accurate Machine Learning models are black boxes as their reasoning is not interpretable by users. This trade-off between accuracy and explainability is a great challenge when critical operations must be based on a justifiable reasoning.”
Prof. Jacques Klein, Chief Scientist (Associate Prof.)
Head of the TruX Research Group
SnT, University of Luxembourg
Professor Klein’s research team is collaborating with a private bank on building explainability for financial machine learning algorithms. The overall objective is for a human to understand how the software made a decision.
Read open access research paper Challenges Towards Production-Ready Explainable Machine Learning
More about SnT research group TruX